
Plastic Landmine Detection Research
Lead Scientist and Cofounder of Safe Pro AI
President and Cofounder of the Demining Research Community
PhD Candidate at Columbia University
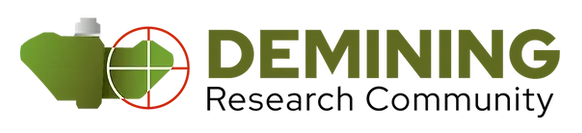
Learn more at
3D model of condensed mine field we buried for research in geophysical detection of buried munitions and UXO.
Scatterable Landmine Detection Research
Through synergies in photogrammetry, thermal imaging, drone platforms and machine learning we have found a new innovative way to detect surface lain PFM-1 anti-personnel landmines. These particular devices pose a difficult challenge to conventional landmine detection methods like metal detecting, because the mines are primarily composed of plastic and only weigh 75 g. As a remnant of the Soviet-Afghan War which lasted from 1979 to 1989, there are an estimated 10 million PFM-1 devices scattered throughout Afghanistan. These mines remain emplaced in isolated locations, frequently out of reach of demining NGOs and act to thwart local economic and social development. The PFM-1s are infamously referred to as “toy mines” as children often mistake the mines for toys and set off the 5-25 kg of cumulative pressure it takes to detonate them.
​
We were able to successfully identify landmines through visual, multispectral and thermal infrared imagery datasets with the Parrot Sequoia multispectral sensor, the FLIR Vue Pro R thermal camera and the DJI RGB camera at 10 meters above ground with various drones. Because the mines have different physical properties like reflectance, emissivity, and thermal conductivity, they heat and cool at different rate than the host geology. In the early morning hours when thermal inertia is greatest, the PFM-1 mines can be detected based on their differential thermal inertia. Because the mine has a statistically different temperature than background and characteristic shape, we have trained a supervised learning algorithm to automate detection of the mines over large areas.
​
With Gabriel Steinberg, we have implemented a Convolution Neural Network (CNN) to automate the detection of scatterable landmines using drones and machine learning. We have created a robust machine learning model that can detect mines in the real-world, and is currently being used to detect mines in Ukraine.
Our goal is the that this technology will aid demining teams in contaminated areas to help save thousands of lives and significantly reduce the cost and time of mine detection, improve safety of operators, and stimulate economic development in these regions.
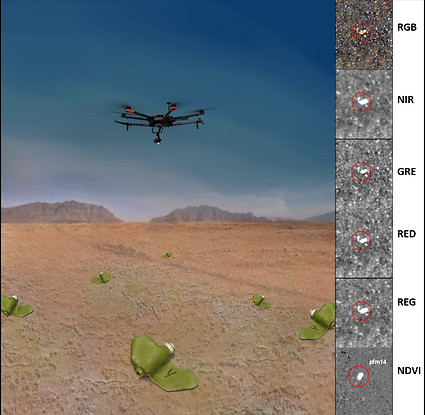
Figure 4. (Baur et al. 2020)
Illustration of experimental design mid-flight in Afghani terrain, using the Parrot Sequoia multispectral sensor attached to the Matrice 600 Pro UAV (unmanned aerial vehicle). Processed multispectral images of the PFM-1 taken from 10 m height during flight.

Figure 1. (Baur et al. 2020)
Rendering of an Inert PFM-1 plastic anti-personal landmine considered in this study with small US coin for scale.